The Future of Object Detection
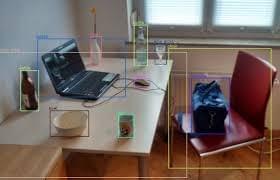
This paper elucidates object detection, one of the highly computational applications that has become possible in recent years. Although detecting objects in a given image or video frame has been around for years, it is becoming more widespread across a range of industries now more than ever before.
Object detection in images and video has received lots of attention in the computer vision and pattern recognition communities over recent years. We have had great progress in the field, processing a single image used to take 20 seconds per image and today it takes less than 20 milliseconds.
Of the problems related to these fields, analyzing an image and recognizing all objects remains to be one of the most challenging ones.
For humans and many other animals, visual perception is one of the most important senses; we heavily rely on vision whenever we interact with our environment. In order to pick up a glass, we need to first determine which part of our visual impression corresponds to the glass before we can find out where we have to move our hands in order to grasp it.
The same code that can be used to recognize Stop signs or pedestrians in a self driving vehicle signs can also be used to find cancer cells in a tissue biopsy.
If we want to recognize another human, we first have to find out which part of the image we see represents that individual, as well as any distinguishing factors of their face.
Notably, we generally do not actively consider these basic steps, but these steps pose a major challenge for artificial systems dealing with image processing.
Most existing algorithms only tackle a small subset of the different tasks necessary for understanding an image and are very expensive computationally. In order to reproduce a fraction of the average person’s ability to detect objects, one would have to combine several different algorithms to make a combined system that runs in real time, an enormous challenge with today’s hardware.
Indeed, object detection is a key task for most computer and robot vision systems. Although there has been great progress in the last several years, there will be even bigger improvements in the future with the advent of artificial intelligence in conjunction with existing techniques that are now part of many consumer electronics or have been integrated in assistant driving technologies.
However, we are still far from achieving human-level performance in open-world learning.
Furthermore, object detection has not been applied in many areas where it could be of great help. Consider for example the possibility of applications of object detection systems to robotic excavation when venturing into previously unexplored territory, such as the deep sea or other planets, in which the detection systems will have to learn new object classes on the job. In such cases, a real-time, open-world learning ability will be critical.
This fascinating computer technology related to computer vision and image processing that detects and defines objects, such as persons, vehicles, and animals from digital images and videos, will be incredibly important in the near future.
We have developed many methods for object detection, but the application of deep learning promises higher accuracy for a wider variety of object classes.
Object detection is breaking into a wide range of industries, including computer vision, image retrieval, security, surveillance, automated vehicle systems, and machine inspection.
Although the possibilities are endless when it comes to future use cases for object detection, there are still significant challenges remaining.
Herewith are some of the main useful applications of object detection: Vehicle’s Plates recognition, self-driving cars, Tracking objects, face recognition, medical imaging, object counting, object extraction from an image or video, person detection.
The future of object detection technology is in the process of proving itself, and much like the original Industrial Revolution, it has the potential to free people from menial jobs that can be done more efficiently and effectively by machines. It will also open up new avenues of research and operations that will reap additional benefits in the future.
Thus, these challenges circumvent the need for a lot of training requiring a massive number of datasets to serve more nuanced tasks, with its continued evolution, along with the devices and techniques that make it possible, it could soon become the next big thing in the future.
Written by Alexander Fleiss
Edited by Gihyen Eom & Calvin Ma