Machine Learning For Investing
Machine Learning For Investing : As data collection and analysis has become more prevalent, the practice of forecasting has risen across many disciplines. From weather prediction to retail shopping, forecasting can be an invaluable practice.
Economists have attempted to forecast market trends and market prices for decades but have been largely unsuccessful.
However, modern advances in data science and machine learning have paved a potential pathway to crisis prevention.
AI Investing
Machine learning models can be used to both predict the coming of a recession and identify contagion risks.
As such these models can not only serve as predictive measures, but containment and damage control in the worst case scenario.
Economists rely on a variety of qualitative and quantitative techniques to predict trends. Experts may use a combination of qualitative practices including surveys, comparing historical resemblances, and market research to arrive at some result [1]. However, qualitative analysis is still largely determinate on opinions and assumption, making it unlikely for a consensus to be reached amongst economists [1].
Although quantitative forecasting techniques are generally subject to less biased than qualitative methods, they can still suffer from poor accuracy. A popular quantitative method is time series analysis using moving averages and exponential smoothing [1]. While it’s a quick and cost effective method of forecasting, it often overlooks variations due to cyclical and seasonal variables [1]. Economic indicators are another commonly used tool in predictive analysis. However, no single indicator can represent the state of an economy and with 120 different indicators available, identifying and combining relevant ones can itself be a challenge [1].
According to Prakash Loungani at the International Monetary Fund, economists have only predicted 2 of the past 150 recessions using traditional quantitative and qualitative methods [5]. Furthermore, prediction accuracy rates have hardly improved over the last 40 years [5]. As the world becomes more interconnected, it becomes increasingly difficult to accurately forecast.
Despite past failures, modern advances in machine learning may be promising. Take research efforts at the University College London. Rickard Nymand and Paul Ormerod developed a machine learning based forecasting model using data encompassing 3 month treasury bill rates, yields on 10 year US bonds, quarterly percentage change on the S&P 500 index, and the ratio of private sector debt to current GDP [2]. The model aimed to predict trends in advance of one quarter with data available to forecasters at the time of interest. Results were quite accurate, see the below graphic:
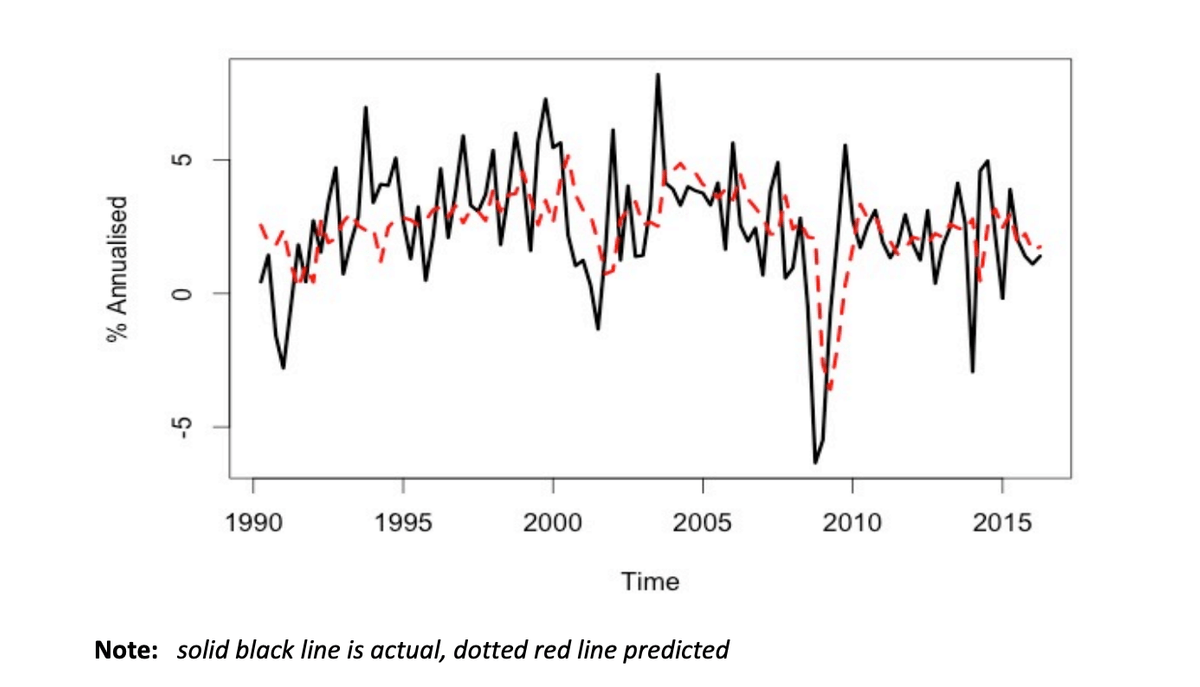
Nymand and Ormerod’s model was able to predict a severe recession in the 2008-2009 time frame and was generally consistent with economic trends from 1990 to 2015. It should be noted that economists were unable to predict the great recession of 2008, with the Federal Reserve and other professional forecasters actually projecting economic growth [4]. The same model was also trained with data specific to the United Kingdom and generated forecasts of comparable accuracy to the above US model.
Machine learning also has the potential to predict contagion risks. Contagion is highly significant in the event of a financial collapse as it can accentuate recessions. Network connections diversify markets’ risk exposure, but they also create channels through which contagion shocks can be spread [3].
Researchers Aristeidis Samitas, Elias Kampouris, and Dimitris Kenourgios at the Abu Dhabi college of business analyzed network connections to build a machine learning model to identify periods of high contagion risk. Samitas et al used weekly stock return data from 2004 to 2016, 10 year bond yields from 2006 to 2016, and 5 years CDS from 2008 to 2016 across 33 different countries to train their model [3].
The model was able to determine periods of high financial contagion and in doing so predicted major recessions including the 2008 subprime mortgage crash and the European debt crisis [3]. Samitas et al also determined various countries’ economies’ interconnectedness in different asset markets [3]. Links between Eurozone, American, and Asian countries were shown in stock, bond, and CDS markets. Analysis even determined insights on specific countries such as French bond markets acting as a central force in european bond fluctuations [3].
Samitas et al’s research in international market connectedness and quantifying contagion spillover can serve as a valuable tool to both policy makers looking to balance world economies and individual investors looking to determine risks and hedge bets. Perhaps if a model similar to their contagion detection system could be applied to a micro setting, the Federal Reserve and other private financial institutions could use concrete quantitative evidence to inform bailout proceedings. Contagion risks are what facilitate the need for federal intervention. If these risks can be accurately predicted, perhaps mistakes like the fall of Lehman Brothers in 2008 can be prevented.
AI Investing
The models discussed above have been reported to have successfully identified past severe economics crises in advance. While each recession is different, successful models seem to use a common set of macro level indicators. Models consistently take advantage of stock indices, 10 year bond rates, and spreads between treasury rates. Perhaps traditional quantitative methods could benefit from these indicators.
While machine learning looks to be a successful alternative to common forecasting practice, economic forecasting is trickier by nature than conventional scientific forecasting. Economic and finance conditions are subject to rapid change and feedback loops [5]. For example, if inflation is reported to rise 3 percent, and wages rise 3 percent accordingly, the situation in which the original forecast was made changes [5]. Now, inflation may rise past what was predicted. ML certainly seems promising but it’s usefulness is proven through hindsight. Hopefully models can be proven through accurately identifying coming economic crises.
Machine Learning For Investing
Citations:
[1] Bob Namvar, PhD. “Economic Forecasting – A Peer-Reviewed Academic Articles: GBR.” Graziadio Business Review | Graziadio School of Business and Management | Pepperdine University, October 30, 2017. https://gbr.pepperdine.edu/2010/08/economic-forecasting/.
[2] Nyman, Rickard, and Paul Ormerod. “Predicting Economic Recessions Using Machine Learning Algorithms,” December 2016. https://arxiv.org/pdf/1701.01428.pdf.
[3] Samitas, Aristeidis, Elias Kampouris, and Dimitris Kenourgios. “Machine Learning as an Early Warning System to Predict Financial Crisis.” International Review of Financial Analysis. North-Holland, May 25, 2020. https://www.sciencedirect.com/science/article/pii/S1057521920301514.
[4]“The Failure to Forecast the Great Recession.” Liberty Street Economics. Accessed February 14, 2021. https://libertystreeteconomics.newyorkfed.org/2011/11/the-failure-to-forecast-the-great-recession.html.
[5] “Why Economic Forecasting Has Always Been a Flawed Science.” The Guardian. Guardian News and Media, September 2, 2017. https://www.theguardian.com/money/2017/sep/02/economic-forecasting-flawed-science-data.
[6] Zhang, Terrence. “Recession Prediction Using Machine Learning.” Medium. Towards Data Science, January 11, 2021. https://towardsdatascience.com/recession-prediction-using-machine-learning-de6eee16ca94.
Machine Learning For Investing Machine Learning For Investing Machine Learning For Investing Machine Learning For Investing Machine Learning For Investing Machine Learning For Investing Machine Learning For Investing Machine Learning For Investing Machine Learning For Investing